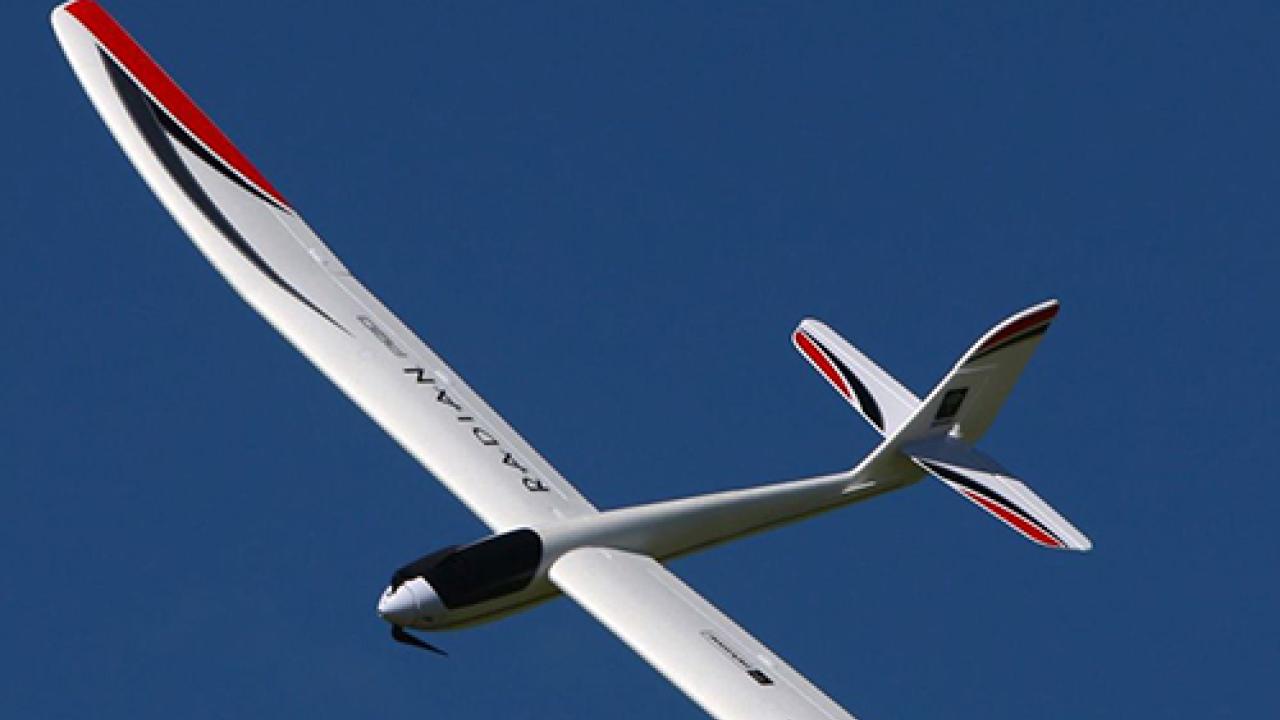
Mechanical gliders can learn to soar like birds with the help of machine learning, reports a study published online this week in Nature.
The research, carried out by ICTP scientist Antonio Celani and colleagues from the University of California San Diego and the Salk Institute for Biological Studies, improves our understanding of how migrating birds extend their flying range and minimize their expenditure of energy, and how autonomous gliders could effectively do the same.
Soaring birds exploit warm, rising air currents — or ‘thermals’ — to fly and gain height without needing to flap their wings. However, the landscape of these currents is complex and continuously changing, and so exactly how birds find and navigate thermals is not understood. Without a full picture, it is hard to teach flying machines to do the same in the field.
In an earlier study, Celani and colleagues shed some light on how migratory birds develop efficient strategies to ride thermals. By combining numerical simulations of atmospheric flow with reinforcement learning methods, the authors identified navigation strategies that could cope with and even exploit turbulent fluctuations, using a learning strategy based on trial-and-error. Also known as ‘reinforcement learning’, this is a dynamic machine learning technique in which an artificial agent learns by interacting with its environment, much like a child, and is ‘rewarded’ for correct behaviour and ‘penalized’ for poor choices.
The research published today in Nature is the result of further investigations of these earlier theoretical findings, using small-scale, remotely controlled gliders for a real-life demonstration of the theory.
![]() |
The authors programmed a two-metre-wingspan glider to adjust its aerial roll and pitch on the basis of on-board measurements of the surrounding environment. By pooling the glider’s collective experiences after a few days of test flights, a navigational strategy was devised, guided by the vertical wind acceleration and roll-wise torque (the force acting to rotate the glider side-to-side) as navigational cues. The authors suggest that the success of this strategy in the field indicates that birds might also rely on such cues.
According to Celani, while the earlier work on developing a numerical learning strategy was crucial to the latest results, the data collected during the glider field trials made for a more efficient learning strategy for the experimental situation. "It was possible to teach a glider this task with a reasonable amount of training time, in the order of few days, which would have been much longer if we had to work by trial and error as we did in the earlier study."
The authors note that being able to soar along an individual thermal is just one part of how migrating birds — or their mechanical mimics — can safely undertake quick journeys across hundreds of kilometres. Future, supplementary research into the navigational cues that could help to identify strong updrafts, they add, will further enhance our understanding of how birds migrate and could aid in the development of efficient long-distance autonomous gliders.
The paper is titled 'Glider soaring via reinforcement learning in the field' (http://dx.doi.org/10.1038/s41586-018-0533-0).