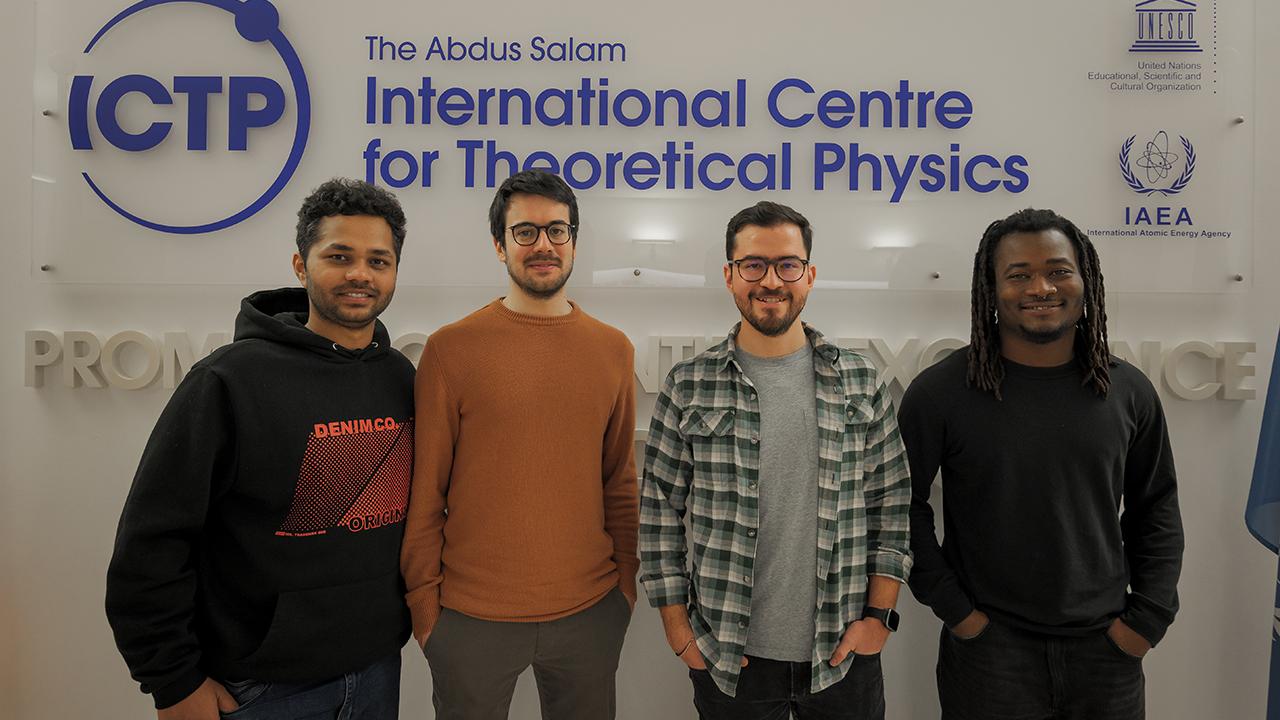
One way in which physicists have dealt with the complexity of reality has been to use abstract thinking and physical intuition to identify the fundamental mechanisms governing observable phenomena. On the other hand, experiments provide us with data so complex that physicists have long needed to rely on models summarizing our theoretical understanding of reality in order to make sense of them.
Thanks to increasingly powerful data analysis tools and the ability to process bigger and bigger data, it has become possible to tackle complexity through a bottom-up approach that applies statistical methods to extract patterns and regularities directly from experimental data, thus opening a new door to understanding complex phenomena.
This is the approach chosen by a group of early career researchers in ICTP’s Condensed Matter and Statistical Physics (CMSP) section, including Roberto Verdel Aranda, Vittorio Vitale, Rajat Panda and Edward Donkor. They are part of a collaboration also involving ICTP research scientists Marcello Dalmonte and Ali Hassanali, as well as an experimental team in Germany, who recently showed [1] that modern data science methods can be applied to extract important information on the universal behavior of out-of-equilibrium systems from raw experimental data, in an approach that requires no assumptions on the underlying system.
Everything started from a challenge: “We asked the experimental group of Markus Oberthaler at Heidelberg University to provide us with some of their data, without telling us anything about how they obtained it,” explains Verdel Aranda, an ICTP postdoctoral researcher from Mexico who first-authored the study. “We wanted to see what our data analysis tools would be able to tell us about the physics behind the data, without any assumption or bias that might have come from knowing what the experiments were about,” he continues.
Before it became a large collaborative endeavour within the CMSP section at ICTP, the project initially involved only Verdel Aranda and Vitale, who at the time had just finished his PhD and was working as a scientific consultant in the section. “Roberto and I started working on the data independently. We wanted to see if we could handle it and extract some meaningful information,” Vitale explains.
“Knowing what the group works on, we could guess that the system was a Bose-Einstein condensate. But only from our independent analysis were Roberto and I able to see that the system was undergoing relaxation from an excited state towards some sort of equilibrium," Vitale adds. “Even more importantly, we were able to see that, while the system was evolving in time, structure was emerging and the number of variables needed to characterize it decreased in time,” Verdel Aranda explains. “Previous work done in our group had shown that this type of pattern is a signature of universality at equilibrium. Our analysis showed that this remains true in out-of-equilibrium systems,” he continues.
“When we shared our results with our experimental collaborators, they became very interested,” Vitale recounts. “They challenged us to see how far we could go in our understanding of the system and if our methods would allow us to obtain better predictions than the ones provided by the methods they usually apply in this context,” he explains.
The initial success ignited excitement in the project and soon more forces were needed in order to dig deeper into the experimental data. Verdel Aranda and Vitale were joined by Rajat Panda, originally from India, who is now a postdoc at the University of Trieste and who at the time of this collaboration was a joint ICTP/SISSA PhD student, working under the supervision of Marcello Dalmonte; and by Edward Donkor, a PhD student in the ICTP-SISSA programme, working under the supervision of Ali Hassanali.
Together, they were able not only to reconstruct information about the initial experiment, but also to define what the important observables in the system were, thus providing their experimental colleagues with a powerful tool that they will be able to use in their future experiments.
As explained by Verdel Aranda, “Much of the interest of our work comes from the fact that, due to the complexity of the systems at hand, experimentalists need to apply theoretical assumptions to interpret their results. Despite working really well, the theories based on these assumptions are only available when we know enough about the system at hand and can use intuition to determine which observables are most relevant.”
But there are other, more complicated systems, which are more challenging to describe theoretically. “Our approach is particularly useful in these cases, to determine what the important observables are,” Verdel Aranda continues. Examples of such systems can be found throughout physics: “systems that undergo topological phase transitions, because there is no local order parameter,” Verdel Aranda says. “In a yet different context, even liquids can be another example of very complex systems,” adds Donkor, who in his PhD applies machine learning techniques to understand the behaviour of water.
It took the stamina of four young and enthusiastic researchers coming from as many different continents to finally tackle the problem and show that modern data science helps us harness experimental data much more deeply than physicists have been able to do so far, thus opening a new door to the understanding of very complex systems.