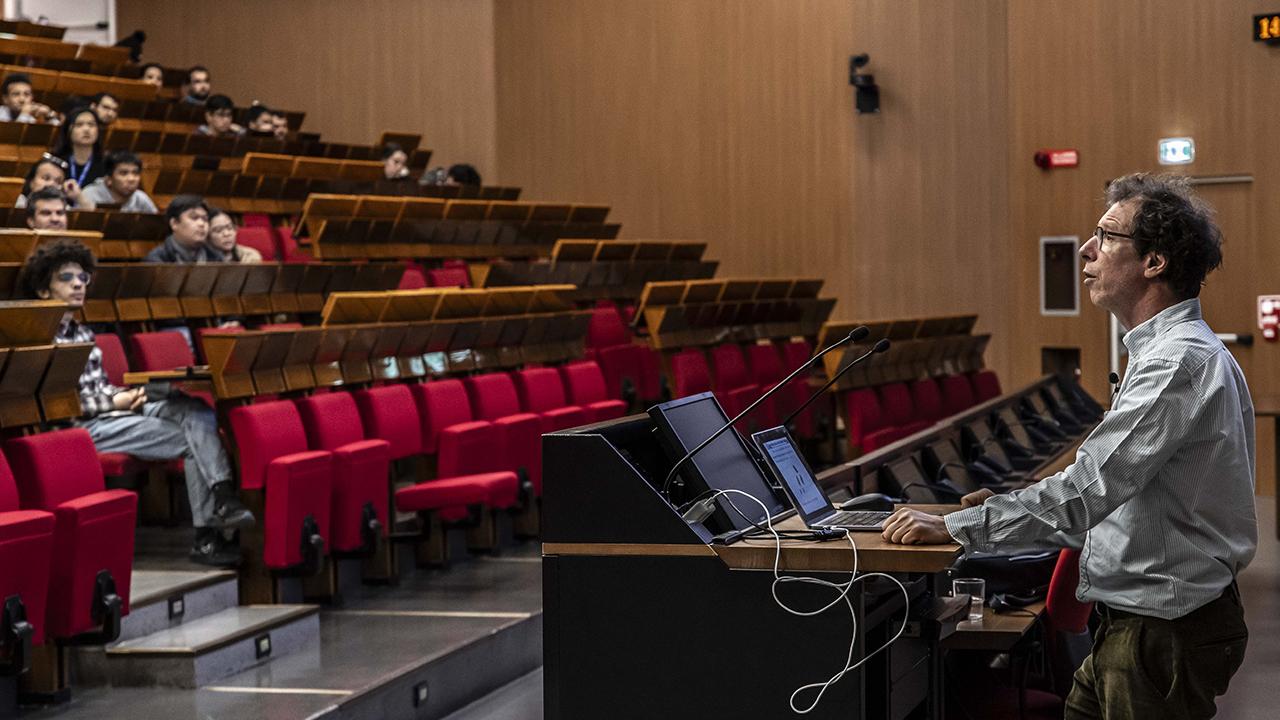
Applied mathematician Stéphane Mallat is an expert in deep learning networks and data science. He is a professor at Collège de France, in Paris, where he holds the Chair in Data Sciences.
After obtaining a PhD at the University of Pennsylvania in the US, Mallat became professor of computer science and mathematics at the Courant Institute in New York, then at Ecole Polytechnique and Ecole Normale Supérieure in Paris. He also co-founded and was the CEO of a semiconductor start-up company.
Mallat’s research interests include machine learning, signal processing and harmonic analysis. Most recently, he has been working on the mathematical understanding of deep neural networks and their applications.
Mallat was recently at ICTP to deliver the 2024 ICTP Salam Distinguished Lectures. Under the theme “Learning Multiscale Energies from Data by Inverse Renormalisation”, Mallat gave an overview of the deep connections between recent advances in data science and theoretical physics, focussing on the Renormalisation group, a paradigm that has been used for a long time in theoretical physics to understand the complexities of phase transitions. In an interview after his lectures, he went back to the topic of his talks. The interview has been edited for clarity.
What are your distinguished lectures about, in very simple terms?
ICTP is a research institute in theoretical physics and in my lectures I wanted to focus on something very interesting going on at the moment in data science and artificial intelligence, that has many points in common with physics. I believe that these advances show the potential for a fertile dialogue, going both ways, between physics and machine learning. Through my talks I wanted to make these bridges evident to the physics community at ICTP because I believe it is important that we exploit these connections in order to advance our knowledge in both fields.
Can you tell us more about this connection between physics and data science?
Until relatively recently, physics was the science dealing with high dimensional problems. What statistical physics essentially does is to study the emergent properties of systems consisting of many interacting particles in order to understand the laws that govern them and predict their evolution over time. Although apparently dealing with very different problems, data science, through machine learning, is nowadays handling very similar questions, at many levels: conceptual, experimental and theoretical.
Let’s start from the conceptual level. Can you explain how physics and machine learning are conceptually dealing with similar problems?
At the conceptual level, machine learning is about analysing large amounts of data in order to recognize patterns, that can be thought of as emerging properties in a system of interacting particles.
Let me explain this through a very specific problem in machine learning: image recognition. In image recognition, the algorithm needs to look at a large series of pictures in order to learn how to recognize and distinguish for example a dog from a cat. In this case your data are images, which are nothing but large sets of pixels. If you think of each pixel as a particle, then recognising different objects in that image becomes equivalent to understanding the interactions between the particles and identifying the structures that these particles form because of the way in which they interact.
Once you formulate the problem in this way, then the connection with physics is very clear: although the domains are very different, it becomes apparent why the mathematics in these fields is essentially the same. It is the same because in both cases the fundamental problem consists in understanding how a large number of particles – or pixels – interact with one another and how these interactions progressively lead to the emergence of specific properties at the macroscopic scale. This is exactly what is at the core of statistical physics, and it helps us understand why machine learning and physics have ended up developing very similar approaches.
Before you explain what happens at the experimental level, could you tell us what you mean by that in the case of machine learning?
Experiments in machine learning consist of developing sophisticated algorithms that are able to learn image recognition and synthesis or natural language processing with great accuracy by training on very large data sets. There have been enormous advances in this domain recently, and machine learning as a field is now essentially driven by experiments.
What is the connection between machine learning and physics at the experimental level?
On the one hand, so far physicists have been making sense of the data coming from their experiments by using models characterized by a certain set of parameters, and by calculating the parameters from data.
However, new, sophisticated technologies such as the Webb Telescope in astrology, for example (but the same is happening in climatology, meteorology and other branches of physics), are providing physicists with more and more data, often referring to systems that are simply too complex to be modeled from first principles. In those cases, one needs to work the other way around, by trying to learn the model from data through machine learning.
Coupling models with machine learning is a strategy that is becoming more and more effective in solving physical problems and there is dialogue going on between physics and machine learning that is opening up new possibilities.
Can you give an example of how machine learning could be coupled with traditional approaches in physics in order to acquire new knowledge?
In meteorology for example, the traditional approach until now has been to solve the Navier-Stokes equations, that describe the evolution of a gas, with initial conditions and parameters provided by actual data at a certain moment in time, in order to predict the weather for the day after. Since in meteorology the parameters change very quickly, it has never been possible to make long-term weather forecasts.
On the other hand, people who work in machine learning would approach the problem differently. In order to make predictions, they do not use models but instead look directly into the data to find what patterns emerge. Despite the large amount of data we have, until recently people were very skeptical that such an approach could work in meteorology, because too many variables are involved. However, about one year ago for the first time, machine learning was used with good performance to predict the weather on a timescale of about 1 or 2 days. Again, it looked like it was not possible to go beyond that limit in time.
New approaches that blend physics with data science are now tackling the problem to allow for predictions over longer time scales. They combine predictions made by machine learning algorithms with models which predict their evolution in time. By going back and forth between these two approaches, one can hope to achieve much better results.
You mentioned the conceptual and the experimental level. How are machine learning and physics connected at the theoretical level?
At the theoretical level, physics and machine learning are connected by the notion of scales.
In both physics and machine learning, the problems we are attacking, be they image classification in machine learning, or the study of many body systems in physics, involve very large-scale phenomena.
In physics, understanding many-body interactions implies taking into account different scales: fundamental particles interact and form atoms, which then form molecules, and molecules make larger structures, which again interact to form even larger structures, and so on, across many scales.
This idea of going across scales in physics is represented and understood through the Renormalization group, a very important paradigm that allows to tackle very complex cross-scale problems by factoring them into much simpler ones. By recursively coarse-graining a globally interacting many-body system, and looking at smaller scale interactions, one can reduce a complex problem into a much simpler one.
The same progressive aggregation of variables can be seen in the architecture of machine learning.
And what does this parallel tell us?
The work that I'm currently doing with my collaborators shows that not only a similar approach can be applied to neural networks, but in so doing it sheds new light on what has been done in physics, where these kinds of tools have only been applied to relatively simple problems.
What we are trying to show is that the new perspective given by machine learning provides for a different interpretation and formalization of the concepts behind the Renormalisation group, through which one can attack much more complex problems. I am thinking for example of turbulence or of the aggregation of mass in the cosmic web, where the interactions are long-range and have so far been impossible to account for in the framework of the Renormalisation group.
What can we learn from these new perspectives?
At the moment there is a very rich exchange going on between data science and physics. Not only are we sharing concepts and applications between these two different fields, but we are also bringing new, important perspectives. It's not just about machine learning gaining a better understanding thanks to tools that were developed in physics, but hopefully also physics can make use of the different mathematical perspective brought by machine learning, to get a deeper understanding of complex physical problems. It is somehow as if the boundaries between machine learning and physics were breaking and cross fertilising both fields.