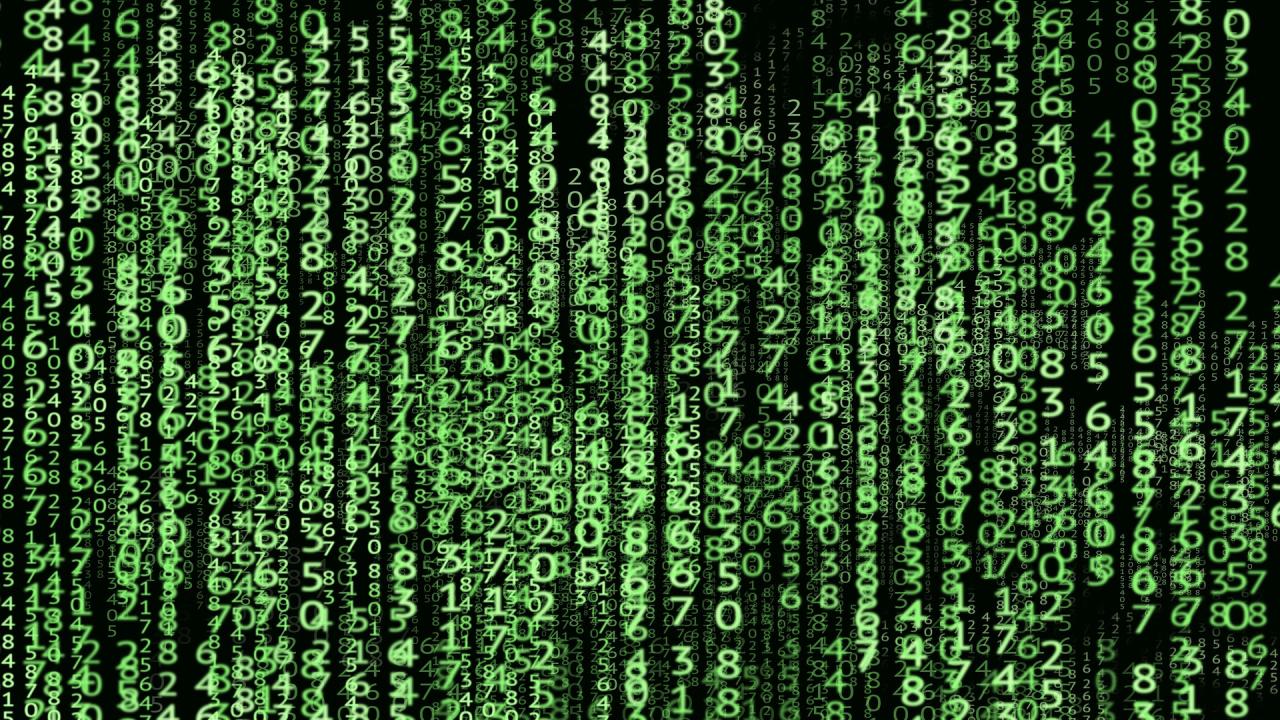
Digital and analog processes that produce signals can be affected by noise. A common task in signal processing is « denoising », namely to extract the signal from the data (the data component of interest, with a structure that is also of interest), and remove the noise (everything else, with a structure that is considered to be of no interest). This means that potentially highly structured information contained in the noise is ignored, which could be used to improve inference of the signal itself.
Research scientists Jean Barbier and Francesco Camilli from the Quantitative Life Science and Mathematics sections at ICTP, in collaboration with Marco Mondelli at the Institute of Science and Technology, Austria and Manuel Sáenz at Universidad de la República Facultad de Ciencias, Montevideo, Uruguay (and who did his Postdoc at ICTP in Barbier’s group), recently published a paper entitled “Fundamental limits in structured Principal Components Analysis and how to reach them” in the Proceedings of the National Academy of Sciences.
The paper describes their research into the role of noise structure, namely the presence of correlations and dependencies within it, when performing signal extraction from corrupted data. This is the first publication resulting from Barbier’s European Research Council grant-funded project [A great opportunity for advanced research in machine learning | ICTP].
The researchers tackled the issue of noise structure by characterising the information-theoretic limits to inference in spiked matrix models. So, what is a spiked matrix model and why were these used in the study? “Spiked matrix models are a class of popular inference problems, used to model the task of low-rank information extraction from noisy data coming in the form of large matrices,” says Camilli. “More specifically, they were introduced to analyse Principal Component Analysis (PCA), namely, the procedure which extracts the few most relevant components (or « directions ») that describe large-dimensional noisy data. This is used in dimensionality reduction and visualisation of complex data, by projecting the data onto few relevant directions that capture most of its variability.”
Previous studies on spiked models often assumed structureless noise represented by a matrix with no correlations between its components, namely, with completely independent entries. “However, we believe this assumption is an oversimplification and far from reality: statistical dependencies exist in the noise, i.e., the data component considered irrelevant for the task at hand. The decomposition of the data into ‘signal’ (the component considered of interest) and ‘noise’ (the rest) is often arbitrary and application dependent,” says Barbier. “For example, in the classification of ‘dogs/cats,’ the training images would contain a lot of information unrelated to dogs and cats, but related to notions such as ‘inside/outside,’ or ‘day/night.’ Yet, this potential source of highly structured information is discarded as random noise.”
Most previous research focused on understanding how signal structure helps inference, whereas much less is known about the role of noise structure and how this may be exploited to improve inference. “We tried to generalize the theoretical framework, uncovering a rich set of new phenomena related to noise structure,” adds Barbier.
There are various applications of the study, which is of particular relevance to high-dimensional data that could contain an underlying low-dimensional or low-rank structure hidden by noise. “One practical example is community detection, where the goal is to identify distinct communities of nodes in a graph based on their connections, such as in prey/predator networks or friendship dependencies in social networks,” adds Camilli. “In such cases, PCA proves effective in estimating the hidden structure of interest, and our work provides an optimal way to consider a much broader class of noises compared to previous literature.”
“We provide bounds to the performance of any algorithm, computationally efficient or not, used for extracting the hidden low-rank information in data corrupted by complex structured noise sources,” says Barbier. The researchers developed the efficient Bayes-optimal Approximate Message Passing (BAMP) algorithm, which provides optimal performance if sufficiently informed about a particular data set. “It intelligently and jointly exploits statistical dependencies within the signal and noise, and can be adapted to different types and strengths of noise correlations,” adds Barbier. “We believe this new understanding will pave the way for new classes of algorithms in various specific application domains where high-dimensional data must be cleaned, such as neuroscience, finance, or particle physics.”
And how does this research contribute to the field of data processing? “We provided the first theoretical universal bound to the performance of PCA procedures when dealing with structured noise,” says Camilli. “Defining the problem itself was a challenge, and prior to our work, the role of noise structure in reconstructing low-rank signals was poorly understood. This interdisciplinary work also produced other findings of interest for use in random matrix theory and statistical mechanics.”
“Our BAMP algorithm can incorporate any prior information about the low-rank signal to effectively exploit statistical dependencies within the noise,” adds Barbier. “It shows superior performance compared to other existing algorithms designed for this problem.”