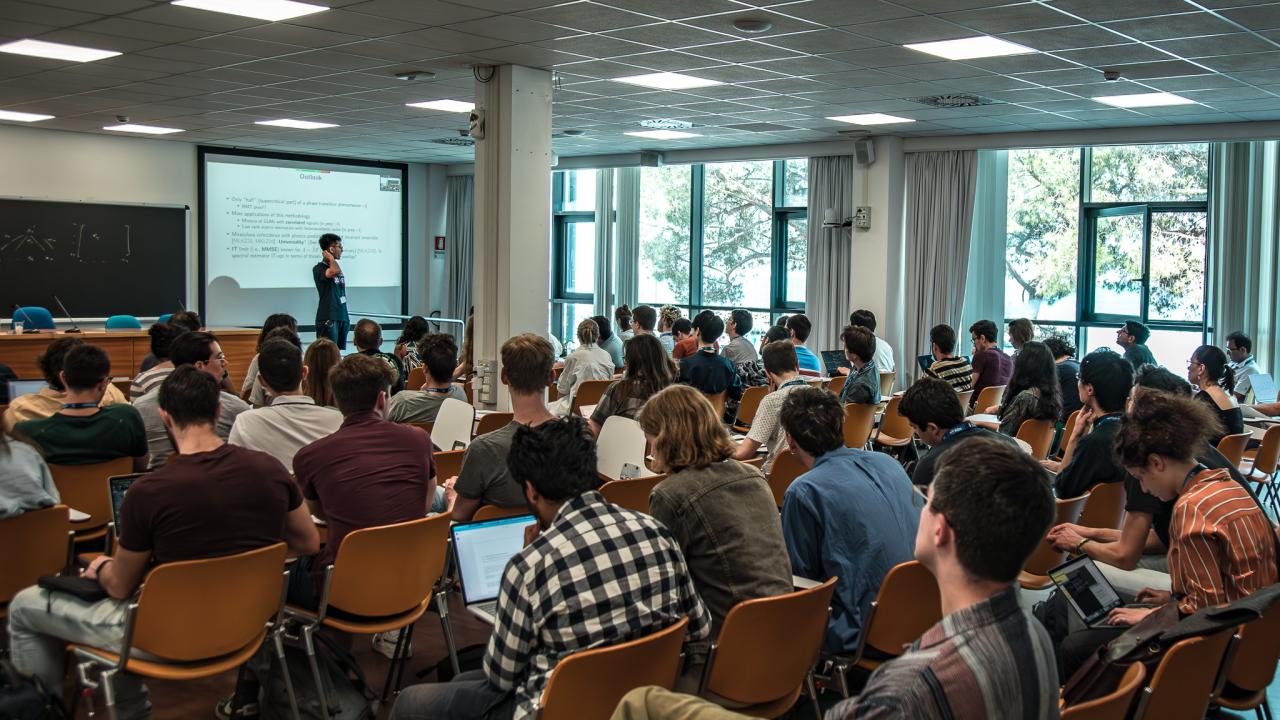
ICTP opened its doors this week for the fourth edition of the “Youth in High Dimensions - Recent Progress in Machine Learning, High-Dimensional Statistics and Inference” event; an array of seminars on high-dimensional scientific problems. The meeting showcased the work of young international researchers from academia and industry on machine learning, high-dimensional statistics and inference. “Youth in High Dimensions” is becoming a key recurring event in a diverse and rapidly expanding community of physicists, computer scientists, mathematicians, information theorists and engineers who study high-dimensional statistics.
“The goal of the meeting is to gather the community at the cross-roads of high-dimensional problems arising in machine learning, inference, statistical physics, and neuroscience, while giving emerging researchers the opportunity to present their results,” says local organizer, Jean Barbier, research scientist with QLS and MATH, who organized the event together with Marylou Gabrié (Ecole Polytechnique, France), Marco Mondelli (IST Austria) and Sebastian Goldt (SISSA, Italy). “So, basically, we only give the floor to young researchers (from end-of-PhD to young faculty) to present their work in a highly interdisciplinary field.”
Scientists from across the world travelled to the event to present and learn about the latest developments in this quickly evolving field. The meeting covered machine learning, signal processing, theoretical physics (quantum systems, spin glasses), statistics and probability (analysis of learning systems), random matrix theory, the analysis of inference and learning algorithms. Throughout the week, seminars focused on such diverse topics as, for example, neural networks, the effect of computer-assisted reasoning on mathematical sciences, free probability in mesoscopic quantum systems, data-centric artificial intelligence, transformers, machine learning-assisted Monte Carlo simulations, and the analysis of stochastic gradient descent.
But why is it so important for researchers across a range of fields to study machine learning, high-dimensional statistics and inference? “Basically, all scientific disciplines are now connected in some way to machine learning, including the most fundamental fields (particle physics, pure mathematics, condensed matter physics, neuroscience, etc.),” replies Mondelli.
Seemingly unrelated high-dimensional problems such as noisy signal estimation, deep neural network analysis, and community detection in large networks have similar phenomenologies, and similar approaches are used to address them. Artificial intelligence approaches such as machine learning provide scientists with new ideas and techniques, which may be used to streamline and develop computational processes.
The feedback between machine learning and scientists shines light on the workings of the method itself. “Machine learning brings new ideas and techniques to all fields of science and vice-versa: scientists from a range of disciplines are now obtaining new insights into why machine learning is so successful,” says Gabrié.
“There is obviously a key economic factor nowadays,” adds Goldt. “A scientific revolution is taking place and machine learning is at the core of it, but there still exists a very large gap between our theoretical understanding of machine learning and its successful applications. This conference is concerned with the theoretical aspects of machine learning, namely high-dimensional statistics (which describes the modern statistics of data science), and is contributing to bridging this gap with the young generation of researchers.”