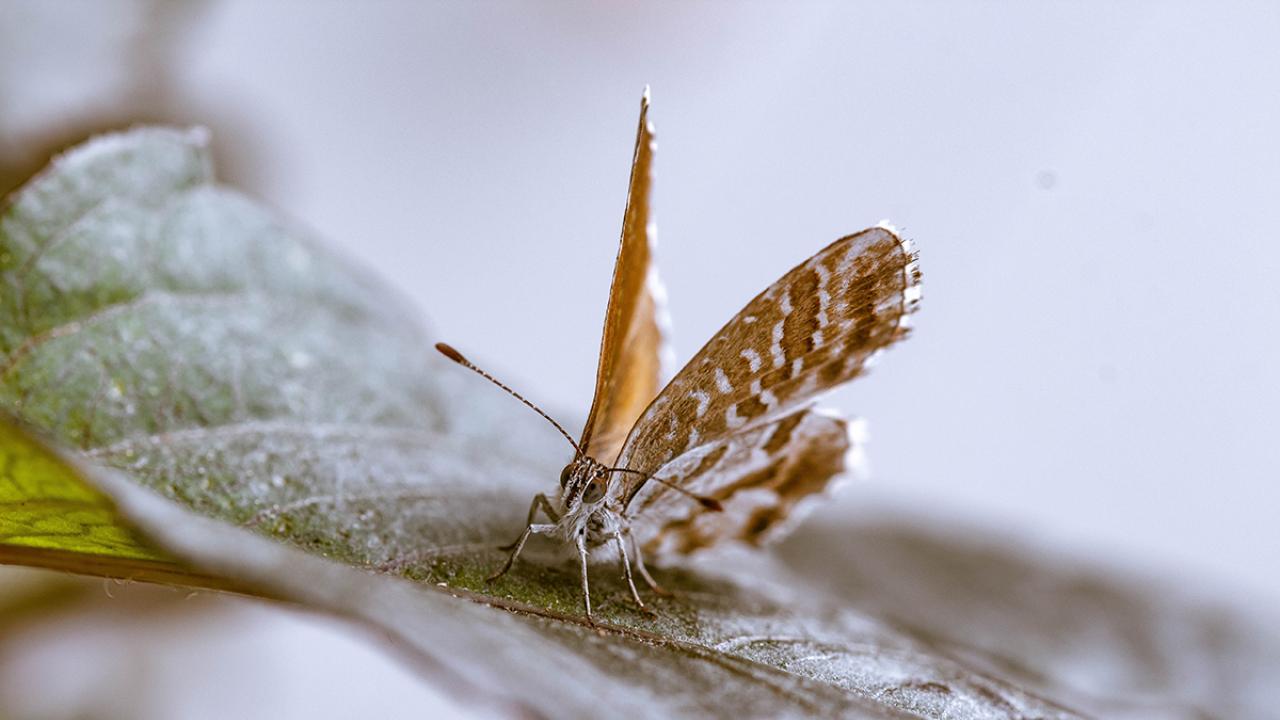
Organisms of all sizes use odor to send information across long distances. Determining the origin of odor is often essential to survival, and organisms such as moths are able to determine the starting point of a sparse odor signal dispersed in the air. However, the sparsity of odors and complex encoding of origin information make it difficult to develop simple search algorithms that exploit this natural phenomenon.
Researchers Antonio Celani, Emanuele Panizon and Kyrell Vann B. Verano of ICTP's Quantitative Life Sciences section developed a computational olfactory search model using algorithmic devices with a small set of discrete memory states. Their model can explain the occurrence of key behavioral patterns observed in nature, according to their recently published paper in the Proceedings of the National Academy of Sciences (PNAS). The group discuss their research, and where it could go in the future.
When did scientists first study natural odor searching processes?
Antonio: In the beginning of the 20th century, a French scientist named Fabre who studied moths kept a female moth in a cage. The story goes that his son noticed there were plenty of moths around the house, and Fabre realized they were male moths, there to mate with the female. The initial limiting step for a male moth is to locate the position of a female by scent, but at the time, no-one had any idea about communication with pheromones.
Experiments in the 60s and 70s later showed that some moths can pick up the scent of a female from hundreds of meters away. This is extremely impressive, because these are very small insects; they produce something like a nanogram per hour of odor, which is then diffused in the atmosphere and mixed with other odors. These moths can pick up one molecule of pheromone in a litre of air.
“We try to understand, from the algorithmic perspective, how some insects are able to search in a complex odor landscape.” - Kyrell Vann B. Verano, PhD student with QLS
What’s your paper about?
Kyrell: We try to understand, from the algorithmic perspective, how some insects are able to search in a complex odor landscape, just guided by odor. Usually, the signals are very intermittent, and we want to understand how they use these intermittent signals to find a source.
Emanuele: I’m focusing on the idea of how memory enters this process, which is all framed inside the field of machine learning.
A: This paper falls at a crossroads between different disciplines. Part of it is about algorithms; a mathematical viewpoint on decision theory. Part of it has a biological motivation, which is inspired by some very interesting animal behaviour. We think this sheds light on possibilities for robotics applications, and has implications for neuroscience.
The guiding principle is that if something is very important in biology, there is a very strong evolutionary pressure for this behaviour to emerge. It has to be done optimally, to guarantee the survival and progression of the individual and species. The optimal solution might be something that has been selected by evolution.
Which methods do you use?
E: We frame the problem of olfactory searching into a mathematically different problem which is much easier, and then solve that smaller problem with computation. We write codes, do simulations, and obtain numbers. The methods we use are generally called reinforcement learning, which is the problem of finding optimal solutions to a task. Entities can perform different actions, and you optimize what kind of actions they do with respect to an overall reward function, which tells you whether the outcome is good or bad. We use these methods to develop a closed form of the olfactory problem, which is clearly biological and extremely complex, into something more manageable. We then apply numerical simulations to that core problem.
We have no direct contact with biologists, but we do exploit experimental data. The problem of olfactory search starts and ends in nature. Reinforcement learning is about strategies and behaviours, which are very hard to infer from experiment.
What's new about your paper?
K: The use of finite simple objects to express past observations. Previous algorithms created a kind of map, which takes up a lot of memory, because you have to store all the pixels in the map. We use very simple controllers as a kind of memory. These are easier to use as a searcher.
E: In our approach, we have few selected behaviours, which are very self contained. There’s this combination of small memory space and quite interesting actions within this memory space.
A: I think the point is this notion of simplicity. We’re using the simplest possible devices, and we could have easily failed, in the sense that this could have shown a performance that was just terrible.
One of the key messages of this paper is that we are looking for simple memory structures. Not only to save computational power, but also because we believe there is no need to look into very complex structures. The line of thought of the paper is that these kind of problems are very old problems in decision making in biology, about how animals pick up scents, and decide where to go based on scent.
What are the limitations of your work?
E: More complex tasks outside our simplified environment may be quite hard to solve. Another limitation is one we are now solving by proposing a memory structure, but then we have to optimize the parameters that define this to solve an actual numerical task. The process of learning the parameters takes a long time computationally.
What are the possible applications of your research?
K: A good application could be in sniffing robots, and to look for chemical leaks.
“We think this sheds light on possibilities for robotics applications, and has implications for neuroscience.” - Antonio Celani, research scientist and head of QLS.
A: In robotics, olfactory search is very important; both in finding gas leaks, and minesweeping. For minesweeping, you can use rats or dogs, but apart from the cruelty of it, it's also extremely expensive, because you have to train them. You really don't want to lose them. Robots are an alternative; you can use robots which just go on the ground, or drones. This approach could become useful there. However, we are not doing any research related to military issues.
What will you do next?
A: One future direction is to use this tool as we have it to address different situations. So, we could use the same approach to describe how bacteria follow chemicals. It's an entirely different scale, and entirely different physics, but the principle is there. So you could ask yourself how a bacterium in the soil moves to reach for nutrients. You'd have to change the models quite significantly, or change the environment, as you change the surroundings, the kind of perception that the organism has, and the way it moves. But, in the mathematical model, these are tuneable parameters. You have the structure of the method, and can apply it to any kind of decision problem.
The second axis of future research is neuroscience. So far, we have been able to explain behaviours with very few memory states. Suppose you have the means to increase gradually the size of your memory, rather than having three or four memory states, now you have 10 or 100. How will the optimal solution shape this network of states? Will it become a chain, in which you jump from one state to the other, will it be branched out, or just a very complex, interconnected network. Neuroscientists are interested in this to predict network structures starting from the function. For this, we have to develop tools that are computationally more efficient, because scaling up is hard. We’re working on this with the High Performance Computing Group here at ICTP.
E: We’re currently trying to see whether our simple memory structure and behaviour linked to the memory are able to reproduce the real behaviour of bacteria or other experimentally known data. What we showed in this paper is that, if we are given the task of finding the origin of odor, we are able to find a good solution. Now the problem is reversed: given a mass of experimental data for bacteria, the question is whether or not we are able to reproduce their behaviour.
Link to the paper: https://www.pnas.org/doi/epdf/10.1073/pnas.2304230120